Data as a driving force of business is being generated more than ever before. Statistical analysis and data mining helps to interpret data and put it to use. With statistical knowledge, professionals and business owners can make sense of data, project future outcomes, and make more informed decisions. Business analytics examples show the power of statistical analysis methods to highlight valuable results and reveals relationships. For example,
Hypothesis Testing
A manager might conduct a hypothesis test to answer the question whether launching a new product line in his/her company leads to increasing sales and revenue as a result. Since it would be so expensive, the company might launch the product in a small test market as a sample and by hypothesis testing expand the results to the company and whole market.
Using Linear Regression for Forcasting
Linear regression analysis can be considered to find the causal relationship between advertising spend and revenue. A business can use historical data relating the advertising dollars spent to the amount of revenue generated for various campaigns or time periods to forecast revenue for future campaigns. Also, instead of looking at total advertising expenditures, multiple regression analysis can be used to evaluate how different types of campaigns, such as television, radio, and social media ads, impact revenue.
Improving HR metrics with statistics
A company might seek to understand how fostering face-to-face interactions among staff can boost employee performance and save money. Microsoft tested this hypothesis, using statistical methods. The findings showed that improving collaboration by moving the 1,200-person group from five buildings to four, which resulted in cutting down on the number of employees per building and reducing the distance that staff needed to travel for meetings, could increase operational efficiency and lead to significant time and cost savings.
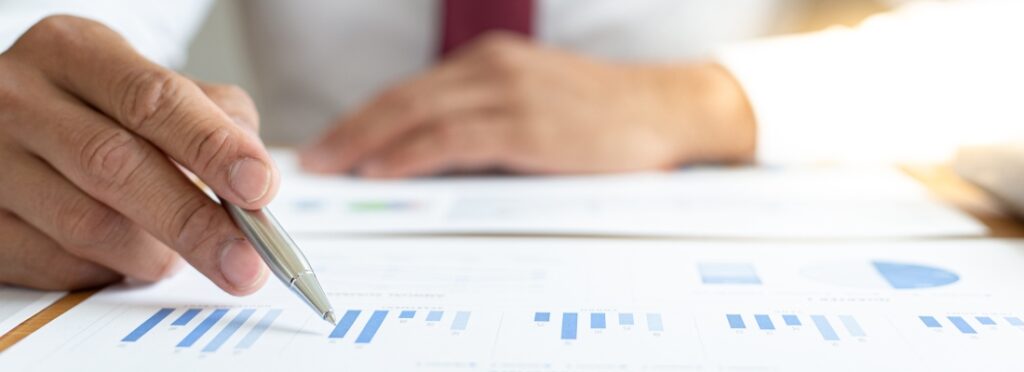
Machine Learning Case Study: Uber
Uber company developed a Customer Obsession Ticket Assistant (COTA) in early 2018 by improving their speed and accuracy when responding to support tickets, applying a tool that uses machine learning and natural language processing. COTA’s implementation delivered positive results. The tool reduced ticket resolution time by 10 percent. For the second iteration of the product, COTA v2, the team focused on integrating a deep learning architecture that could scale as the company grew. Before rolling out the update, Uber turned to A/B testing—a method of comparing the outcomes of two different choices (in this case, COTA v1 and COTA v2)—to validate the upgraded tool’s performance. Preceding the A/B test was an A/A test, during which both a control group and a treatment group used the first version of COTA for one week. The treatment group was then given access to COTA v2 to kick off the A/B testing phase, which lasted for one month. At the conclusion of testing, it was found that the use of COTA v2 led to faster service and more accurate resolution recommendations, which slightly improved customer satisfaction scores. With the use of A/B testing, Uber determined that implementing COTA v2 would not only improve customer service but save millions of dollars by streamlining its ticket resolution process.
Understanding Customer Behavior with Predictive Analytics
For meal kit delivery service Blue Apron, understanding customer behavior and preferences is vitally important to its success. Each week, the company presents subscribers with a fixed menu of meals available for purchase and employs predictive analytics to forecast demand, with the aim of using data to avoid product spoilage and fulfill orders. Customer-related features describe historical data that depicts a given user’s order frequency, while recipe-related features focus on a subscriber’s past recipe preferences. In the case of seasonality features, purchasing patterns are examined to determine when order rates may be higher or lower, depending on the time of year.
Precise Placement with Predictive analysis: PepsiCo
PepsiCo uses big data and predictive analytics to ensure the right quantities and types of products are available to consumers in certain locations. PepsiCo created a cloud-based data and analytics platform called Pep Worx to make more informed decisions regarding product merchandising. With Pep Worx, the company identifies shoppers in the United States who are likely to be highly interested in a specific PepsiCo brand or product. Pep Worx enabled PepsiCo to distinguish 24 million households from its dataset of 110 million US households that would be most likely to be interested in Quaker Overnight Oats. The company then identified specific retailers that these households might shop at and targeted their unique audiences.